With Magnetic resonance imaging (MRI) we can examine brain anatomy noninvasively. The contrast in these images is particularly useful for the detection of abnormalities related to neurological diseases.
For example, one of the most important markers for the diagnosis and monitoring of Multiple Sclerosis (MS) is the presence and volume of white matter lesions in the brain. The detection of such lesions relies on a specific MRI modality that highlights white matter damage, the T2-weighted fluid-attenuated inversion recovery (as shown in the video).
However, it is not always possible to get 3D MRI with good resolution due to technical or time restrictions, so images are often acquired with low resolution, which can make it hard to extract accurate information from them. This is a big problem in MS because image resolution directly affects the detection of lesions and the estimation of their volume.
We are working on developing and testing super-resolution tools that use physics-based modeling and machine learning (aka AI) to obtain a high-resolution brain image from a set of low resolution images. With super-resolution we can get more accurate estimates of lesion volume for MS patients using MRI acquired with low resolution.
Currently, we are particularly interested in the use of super-resolution to facilitate the investigation of image-based biomarkers with retrospective MRI databases containing low-resolution scans, and the use of super-resolution in the development of accessible low-field MRI scanners that could be used at point-of-care facilities.
See the effect of super-resolution MRI
on the segmentation of MS lesions
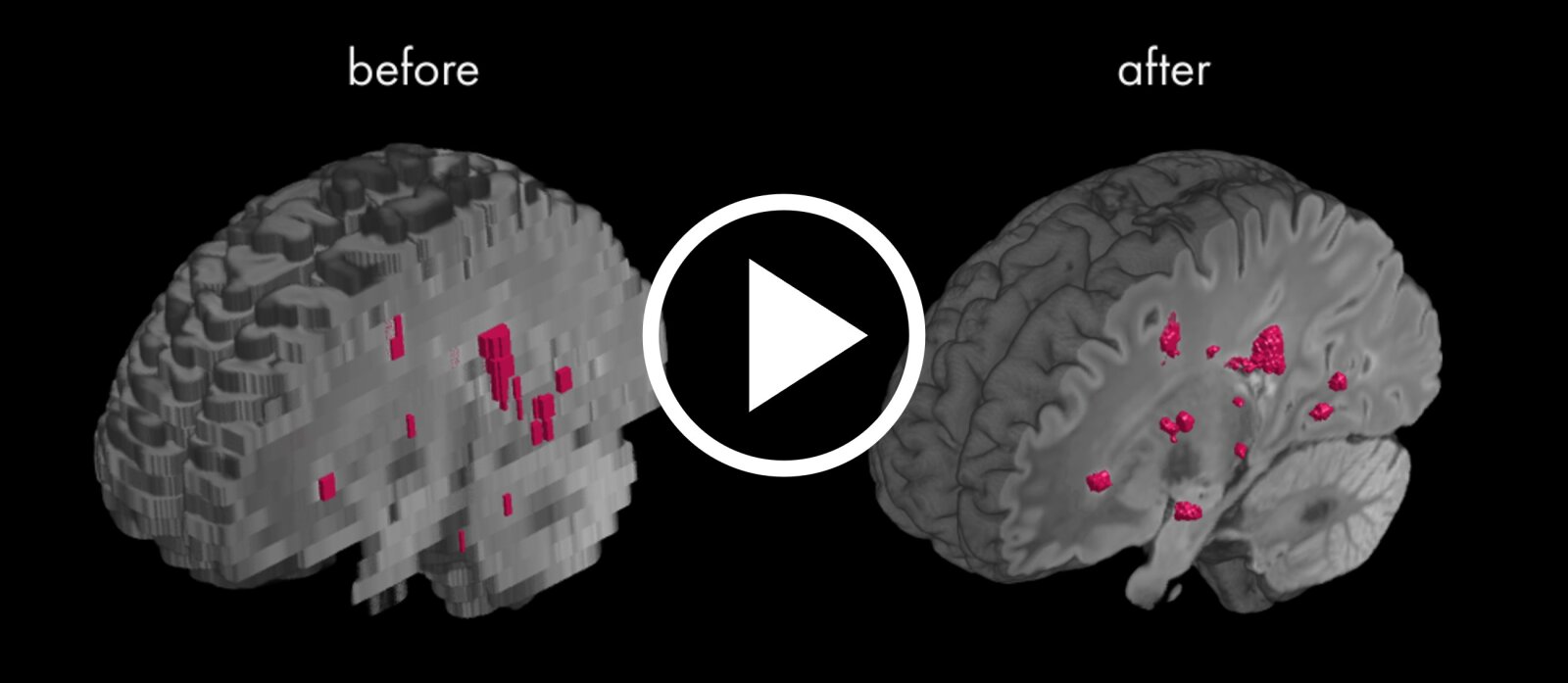