Extraction of high resolution information from coarsely sampled data
Researchers at the University of Antwerp developed a sub-Nyquist regular sampling scheme that delivers superresolution output using a combination of recent results in sparse interpolation and approximation theory.
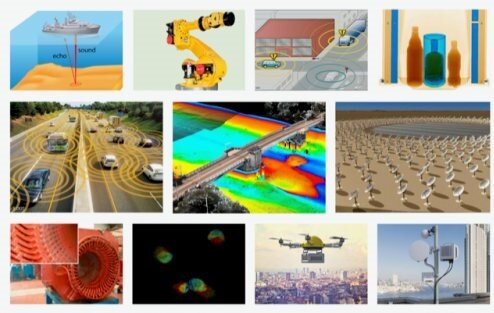
Multi-exponential analysis might sound remote, but it touches our lives in many surprising ways, even if most people are unaware of just how important it is. For example, a substantial amount of effort in the field of signal processing is essentially dedicated to the analysis of multi-exponential functions whose exponents are complex. The analysis of exponential functions whose exponents are very near each other is directly linked to super-resolution imaging. As for multi-exponential functions with real exponents, they are used to portray relaxation, chemical reactions, radioactivity, heat transfer, fluid dynamics.
Multi-exponential analysis is fundamental to magnetic resonance and infra-red spectroscopy, vibration analysis, direction or angle of arrival detection, speech and music signal processing, automotive radars, time series analysis, transient detection, induction-motor fault diagnosis, fluorescence-lifetime imaging, electronic odour recognition, drug-clearance monitoring and glucose-tolerance testing, to name just some of the more well-known examples.
Situation before
Despite their computational efficiency and wide applicability in digital signal processing, Fourier-based methods suffer from some well known limitations, such as a limited resolution and leakage in the frequency domain. They are also not very well suited for aperiodic signals, such as exponentially decaying ones. Multi-exponential analysis on the other hand can offer superresolution but, in practice, may suffer from ill-posedness or ill-conditioning. The underlying method to reconstruct the exponential sum is already much older and goes back to the Prony. Both Fourier- and Prony-based methods make use of the Shannon-Nyquist theorem which states that the minimum sampling rate required to recover a signal is at least twice the maximum frequency present in the band-limited signal.Recent achievements, allowing to circumvent the Shannon-Nyquist sampling rate, regularize the multi-exponential analysis and break the curse of dimensionality in case of higher-dimensional analyses, have unlocked new potentials.
Technology offer
We developed a parametric method to retrieve high-resolution information from coarse-scale measurements, while maintaining the use of a regular downsampling scheme. We exploit, rather than avoid, aliasing in order to regularize the problem statement and increase the resolution.The technique is very versatile because it can be combined with different existing multiexponential analysis methods (matrix pencil,MUSIC, ESPRIT, ...). It seems to be especially useful in the presence of clusters of frequencies that are difficult to distinguish from one another. These one-dimensional existing implementations can also be combined with some structured linear systems for use in the multivariate case and thus realize a multidimensional exponential analysis from the theoretically minimum number of samples, thereby breaking the curse of dimensionality.
About the researchers
The technique is developed in the research group Computational Mathematics, by Annie Cuyt (head) and Wen-shin Lee (postdoc). Computational mathematics involves mathematical research in areas of science where computing plays a central and essential role,emphasizing algorithms, numerical methods, symbolic methods and scientific computing.
IP Position
• Annie Cuyt and Wen-shin Lee. Smart data sampling and data reconstruction. Patent application EP 2745404. Filing Date 20/08/2012. Grant date 21/03/2018.• Annie Cuyt and Wen-shin Lee. Smart data sampling and data reconstruction. Patent US 9,690,749. Filing Date 20/08/2012. Grant date 27/06/2017.